20 Best Product Data Analyst
Freelancers
to Hire
in 2024
Looking to hire Product Data Analyst freelancers with the perfect set of skills for your next project? Work with the world's best freelancing talent on Contra.
Andy Luo
Bridging the gap between data analysis and market
ROHIT PODDAR
Data Analyst: Transforming Insights 📊
ugbaje innocent
Data Analyst / Business Analyst /PowerBI Developer
Braedon Shick
Uncovering the Hidden Treasure in Data
Srikanth Tangirala
Data Analyst / Data Scientist
Nhung Le.T.H
Data Analyst | Nice story now show me the data
Pratik Srivastava
Data Analyst & Visualizer 📊✨
Irfan Sajjad
Data Analysis | Dashboard Creation | Reporting
David Smith
Data Scientist | Business Value from Data 📈
Lauren Brady
Data-driven product marketer for revenue growth 🚀
Nahom Tsehaye
Nahom Tsehaye
Meg Tsevegmid
Data Analysis Expert & Web Developer
Amy Lin
Customer-centric problem solver
Sydney Sauer
Data analysis that wows through design
Andrii H
Senior Data Engineer | Database Architect | AI/ML
Richard Constantine
Agile Product Manager & Data Analyst
Haider khan
Software engineer skilled in data analysis, machin
Kanav Dawra
Data Scientist | Web Developer | Software Engineer
Product Data Analyst related skills for your next project
Hire the best freelancers with ease
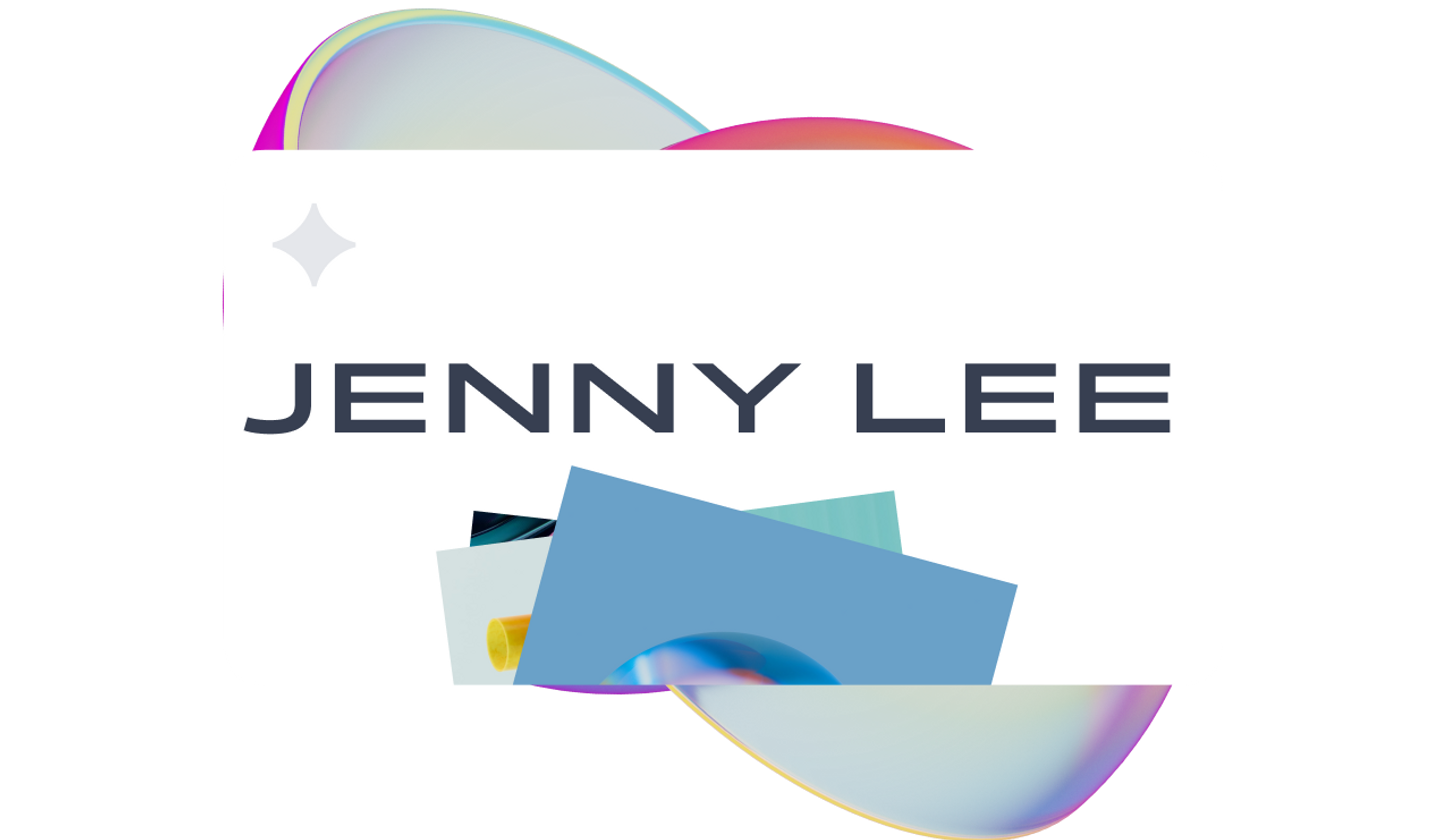
Discover vetted
talent
Connect with verified, high-quality freelancers.
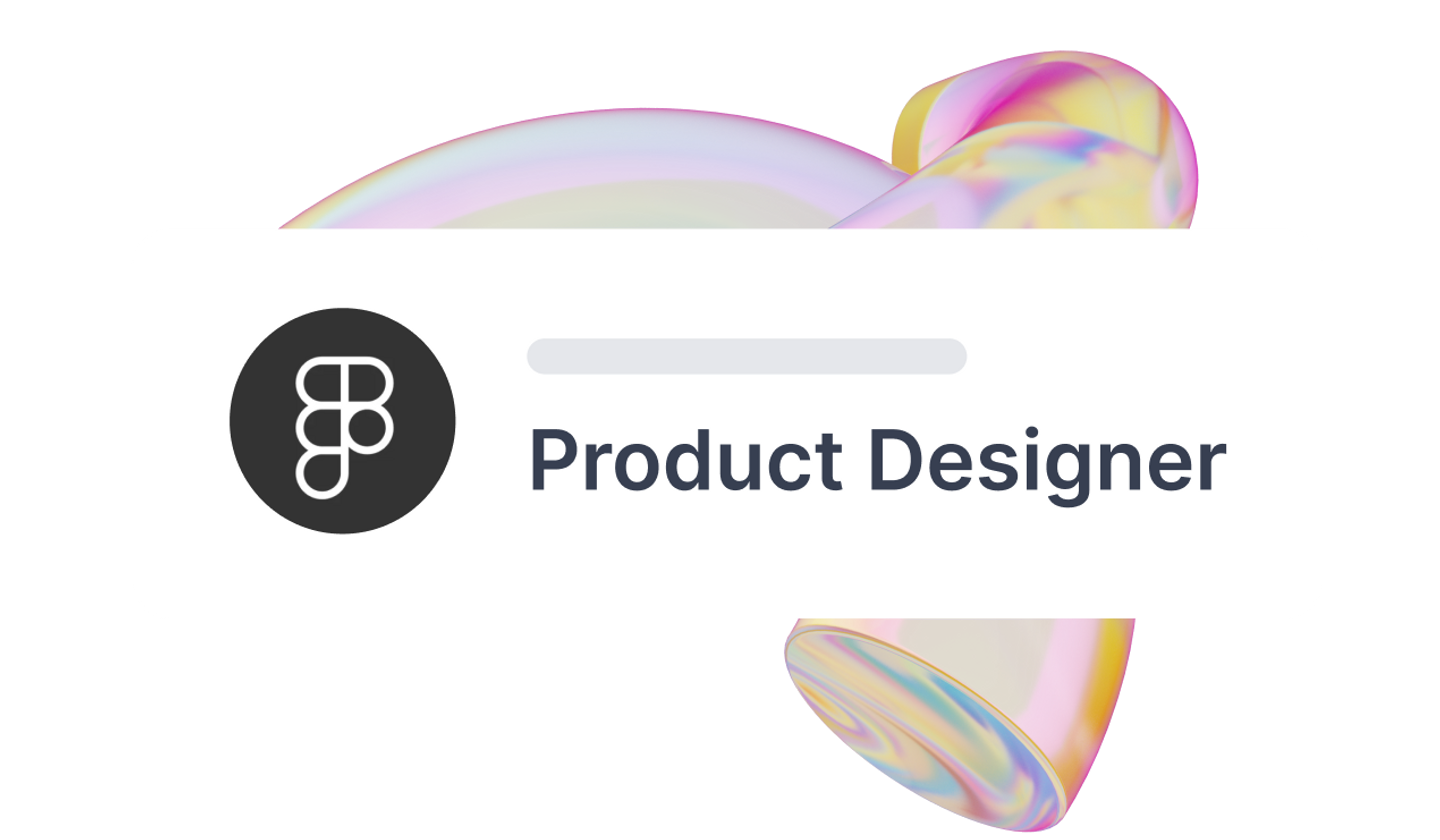
Expert matching for
your opportunity
Post your opportunity and we’ll do the work for you.
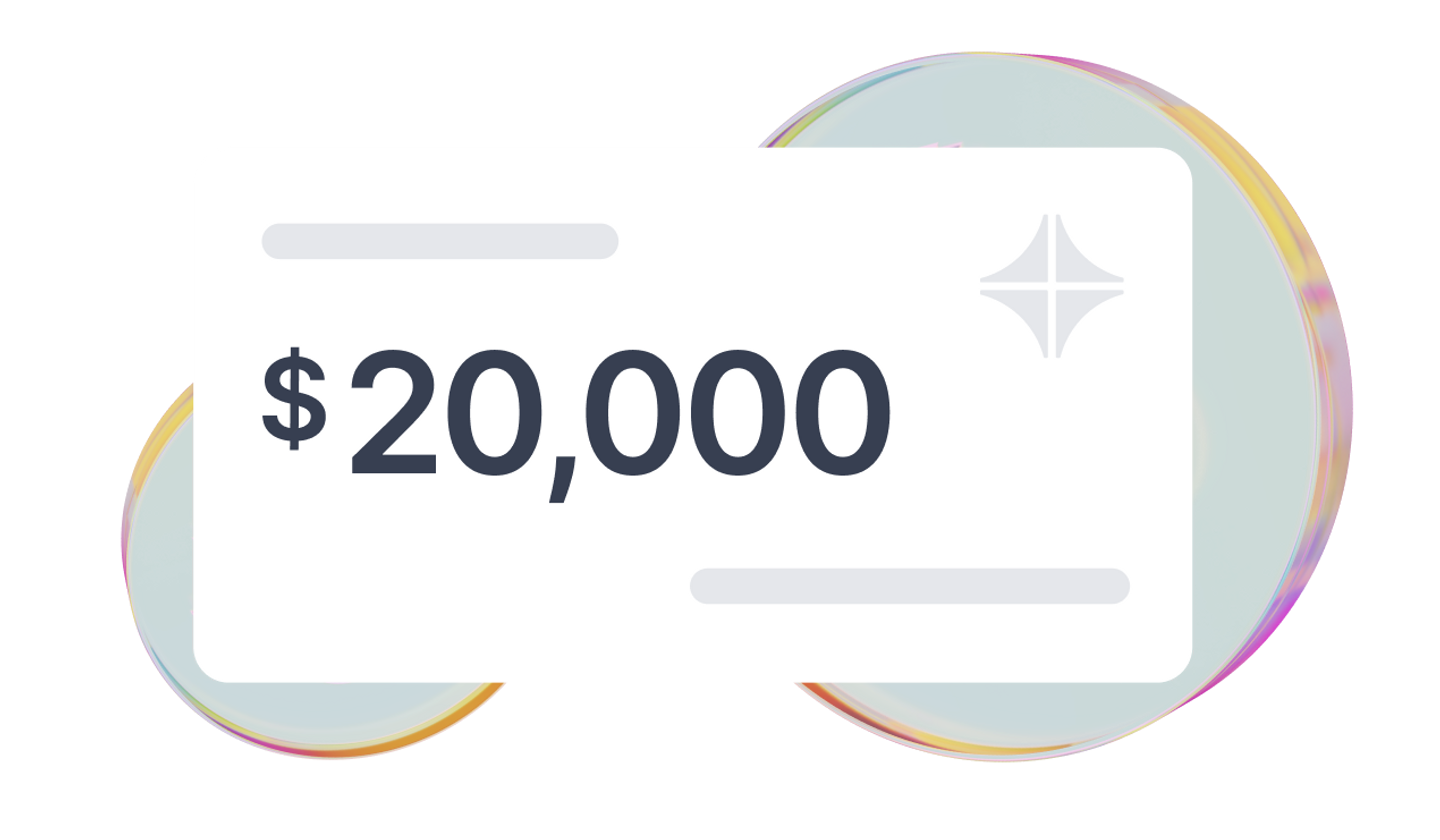
Manage
contracts & payments
Create contracts, pay, and chat with freelancers.
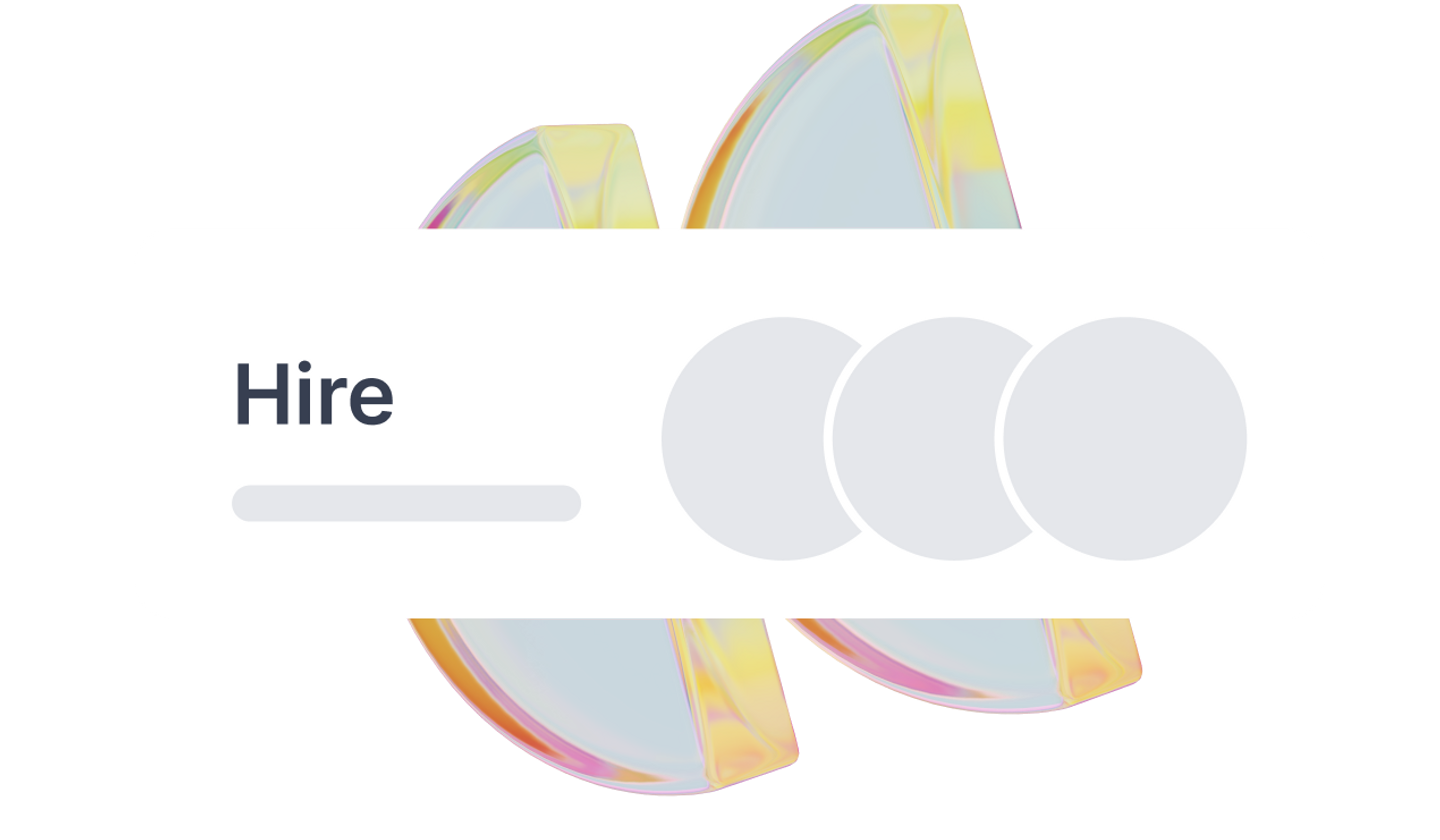
Scale your business
Focus on core business functions by outsourcing.
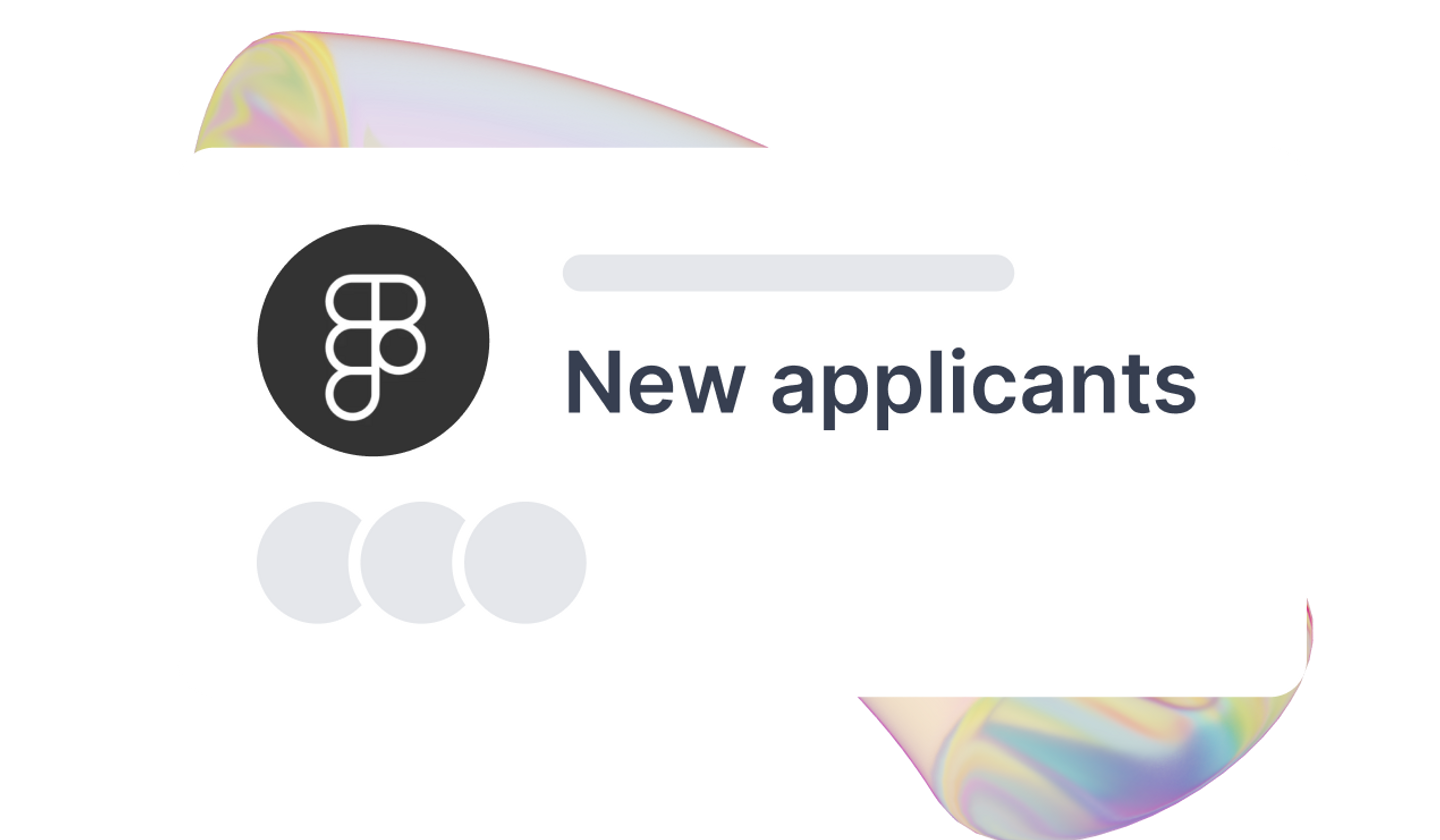
Save time & money
Let our team help you through the process of hiring, all for free.
99% of Clients report having a positive experience on Contra
Why Clients trust Contra
The quality of independents on Contra is top-notch, and the onboarding process is one to model. Being able to speak with a team member to truly understand what we needed as well as offer suggestions of talent in the network was a whole new experience for me.

Love, love, love. Contra is an amazing place to find flexible, creative talent. The process of getting to know independents through their profiles was incredibly easy and fast.

The talent I find on Contra has been excellent. But aside from that, Contra’s community differentiates Contra from other freelancing platforms. I love the responsiveness of the Contra team, and how invested they are in your success. I actually pay freelancers I’ve found outside of Contra through Contra because I love the platform so much, especially that they don’t take a cut of your payment.

I used to waste hours trying to find talent. Switching to Contra was 10x on my time, energy and money as an Entrepeneur and Creator. Lauren was a perfect fit from day one, and I couldn’t have found someone of this calibor without Contra.

I can vouch for Contra that it is a great hiring tool. I shoot my shot and the candidates I keep seeing are incredible.The process took minutes and the support I always get from their team is 10/10.

Freelancers on Contra come across very professionally. They actually seem to read your job posting and have directly relevant skills, which isn’t always the case on most other freelancer sites. On Contra, freelancers who matched with my job seemed to have the relevant skills I was seeking.

How to get started on Contra
Contra for Clients
With Contra, you can discover and hire top-notch talent, manage payments, contracts, and projects, and work the way you want.
Read moreGuide to Discover
This guide will provide the steps to finding your perfect match on Contra using our Discover tool
Read moreHow to Post a Top-Tier Opportunity
If you need an Independent for your next opportunity, you're in the right place. Learn about how you can find your next collaborator.
Read moreHow to hire on Contra
There are three ways to hire on Contra -- we’ll go through all three of these in this article and when to use each one!
Read moreBest Practices for Working with Independents
Thinking about hiring an Independent? Here are some tips to maximize your partnership and collaboration
Read moreLearn more on hiring
Explore the Blog
Related articles
Data Scientist vs Data Analyst: Key Differences ⚖️
- Engineering, Dev & IT
How To: Become a Freelance Product Manager with Abidal Alshahri
- Remote Working & Freelancing Design & Creative
What Is Talent Acquisition? Unveiling Strategies for Success 🔎
- Remote Working & Freelancing
What Is Data-Driven Marketing? Everything You Need to Know 📊
- Marketing
Cost-Benefit Analysis: What It Is & How to Do It Like a Pro ⚖️
- Remote Working & Freelancing
Mastering the Art of Creating a Social Media Content Strategy📱
- Social Media