20 Best Data Engineer
Freelancers
to Hire
in 2024
Looking to hire Data Engineer freelancers with the perfect set of skills for your next project? Work with the world's best freelancing talent on Contra.
Christine Straub
Full-Stack AI/ML Software Engineer
Asif Farhan Khan
Data Engineer | Cloud & Python Developer
Jonathan Williams
Full Stack Developer & Data Scraping Expert
Abhiram Kannuri
Python Expert: Web Scraping & Automation | Lead Data Analyst
Subrin Al Azad
UI/UX Designer comes with programming skill
Ian Wilson
Software Engineer and Technical Leader
Bilel Guembri
Data Extraction Specialist
Lucinda Beeson
Data Analytics, Engineering and Automation
Wamaitha Nyamu
Software Engineer
Malik Fasih
AI Chatbot Developer | Fullstack Engineer
Shubhashish Dixit
SQL Data Visualization Tableau Power BI Metabase
Bogdan Drema
Anything's possible, just a question of time
Francisco Perez
Business/Data Analyst
Jakub Dabkowski
Senior Data Engineer
Kaustubh Bhiwsankar
Data Analytics Professional
Michael Stoeckl
Data-baacked Conversion Optimization for ECommerce
Paolo Perrone
AI + Data Copywriter & Consultant
Pavel Jeลพek
Transforming Data into Business Success
Uche Ogbuji
Pioneering AI/Data/Software engineering leadโ Builder/solver
Data Engineer related skills for your next project
Common projects for Data Engineers
Building and optimizing data pipelines, architectures, and data sets.
Designing and developing data applications using selected tools and frameworks.
Implementing ETL processes and data warehouse architecture.
Managing and designing the reporting environment, including data sources and security.
Improving data foundational procedures, guidelines for data collection, and data sharing.
Creating data tools for analytics and data scientist team members.
Tips for working with and hiring Data Engineers
Understand your data needs
Before you hire a data engineer, make sure you understand your data infrastructure needs. This includes the type of data you will be working with, the scale of data, and how the data will be used.
Review their technical skills
A data engineer must be proficient in databases, ETL tools, and programming languages. Make sure to review their skills in these areas before hiring.
Look at their experience
Experience in relevant industries or projects can be a huge plus. A data engineer with experience in similar projects or industries will be able to bring valuable insights to your project.
Communicate effectively
Regular and clear communication is vital for the success of any project. Make sure to provide clear instructions and be open to discussions and suggestions from the engineer.
How to hire Data Engineers
Define your needs
Before starting the hiring process, have a clear understanding of what you need from a Data Engineer. This could include data infrastructure setup, ETL process design, or data quality assurance.
Post a detailed job
Posting a detailed job on Contra will attract the right freelancers. Include information about your company, the scope of the job, the skills required, and the project timeline.
Review applications
Review the applications you receive and shortlist freelancers based on their skills, experience, and fit for your project. Look at their past projects and reviews on their profile.
Conduct interviews
Conduct interviews with shortlisted freelancers. Ask questions about their experience, approach to data engineering, and how they handle various aspects of data engineering. Also, discuss your project specifics and expectations.
Make an offer
Once you've found the right freelancer, make an offer through Contra. This will include the project details, payment terms, and timeline.
3 questions to ask when interviewing Data Engineers
1. Can you describe a project where you designed and set up a data infrastructure from scratch?
2. What experience do you have with ETL processes and data warehouse architecture?
3. How do you ensure the quality of data in your projects?
Hire the best freelancers with ease
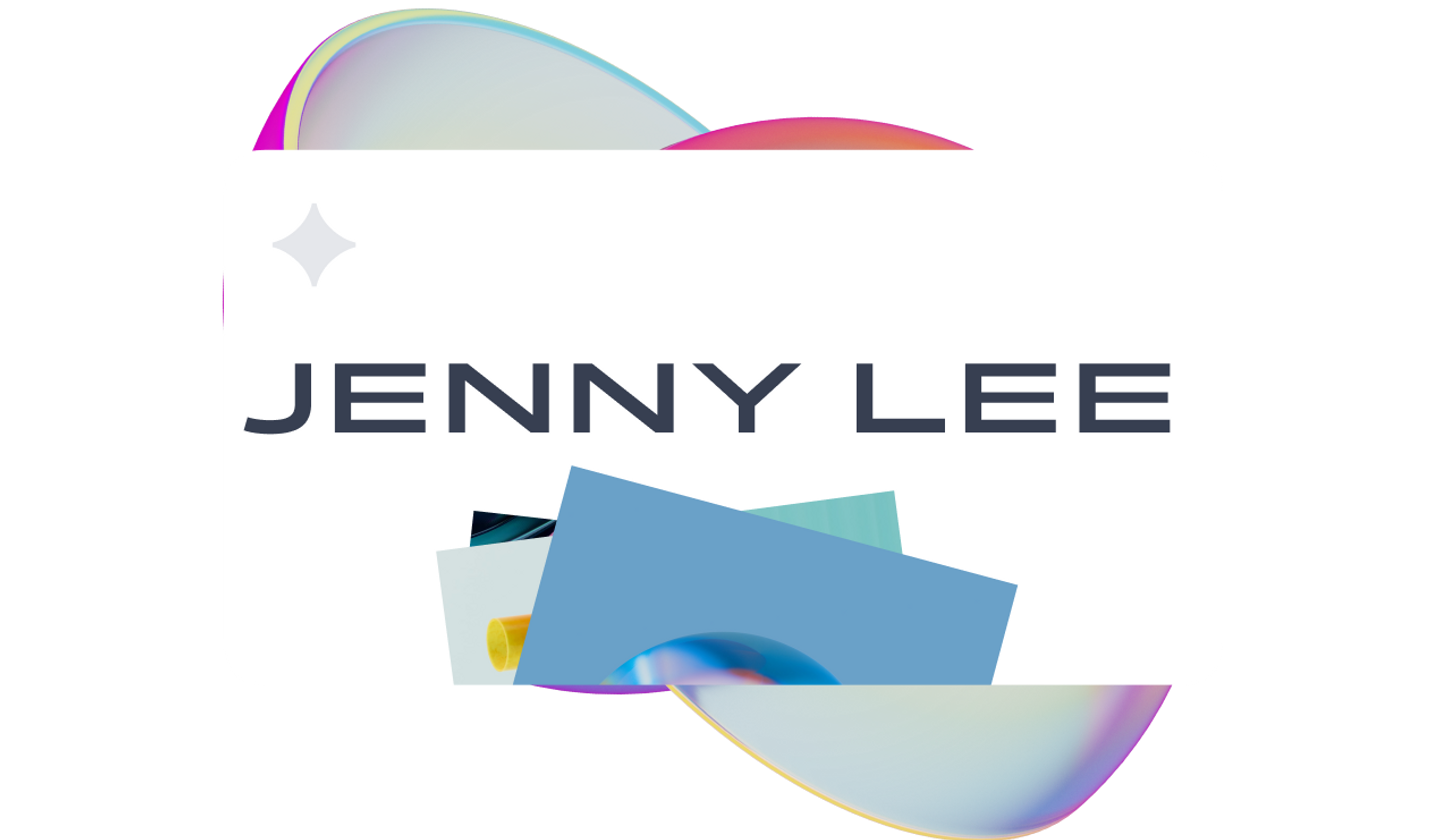
Discover vetted
talent
Connect with verified, high-quality freelancers.
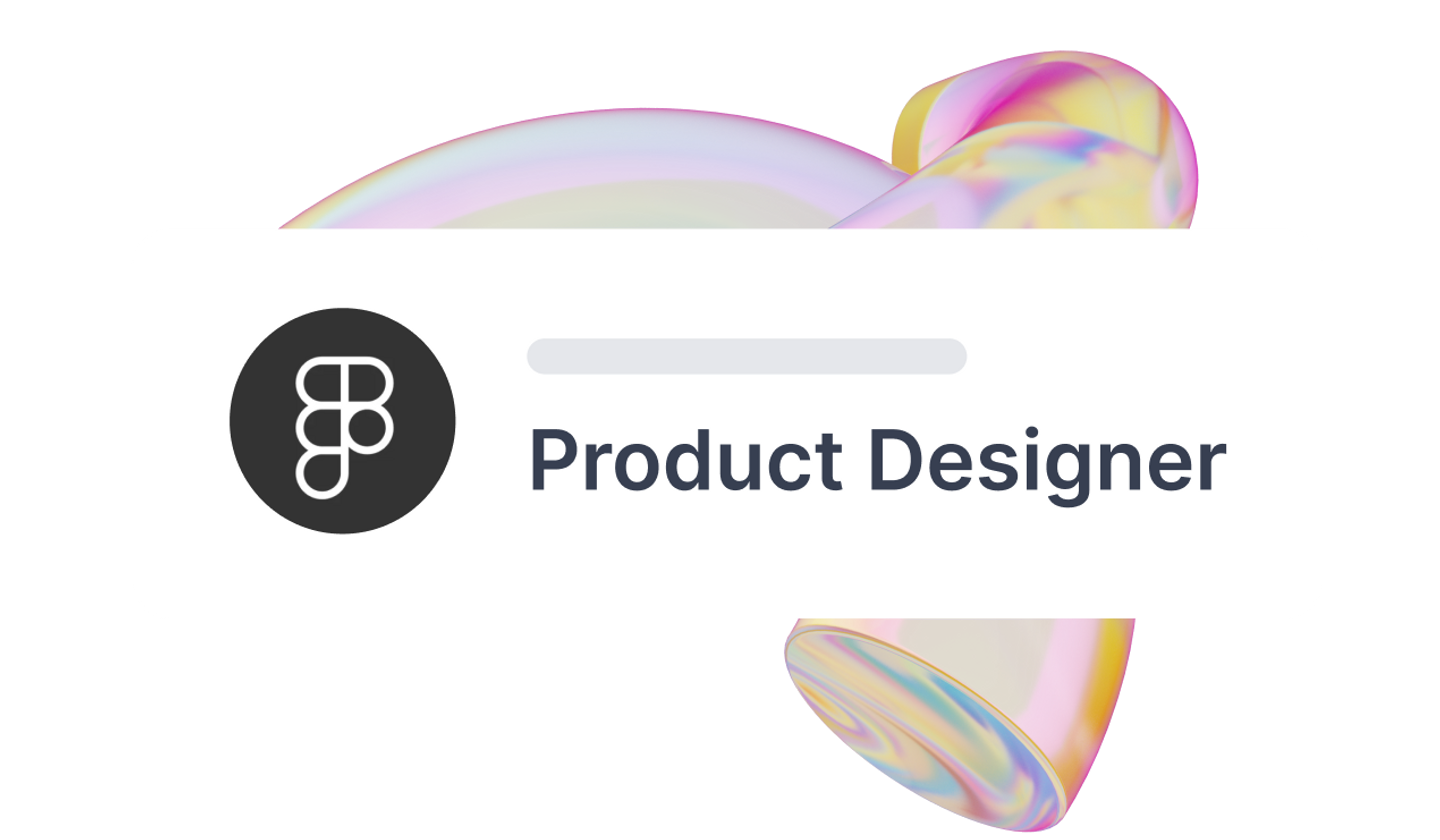
Expert matching for
your opportunity
Post your opportunity and weโll do the work for you.
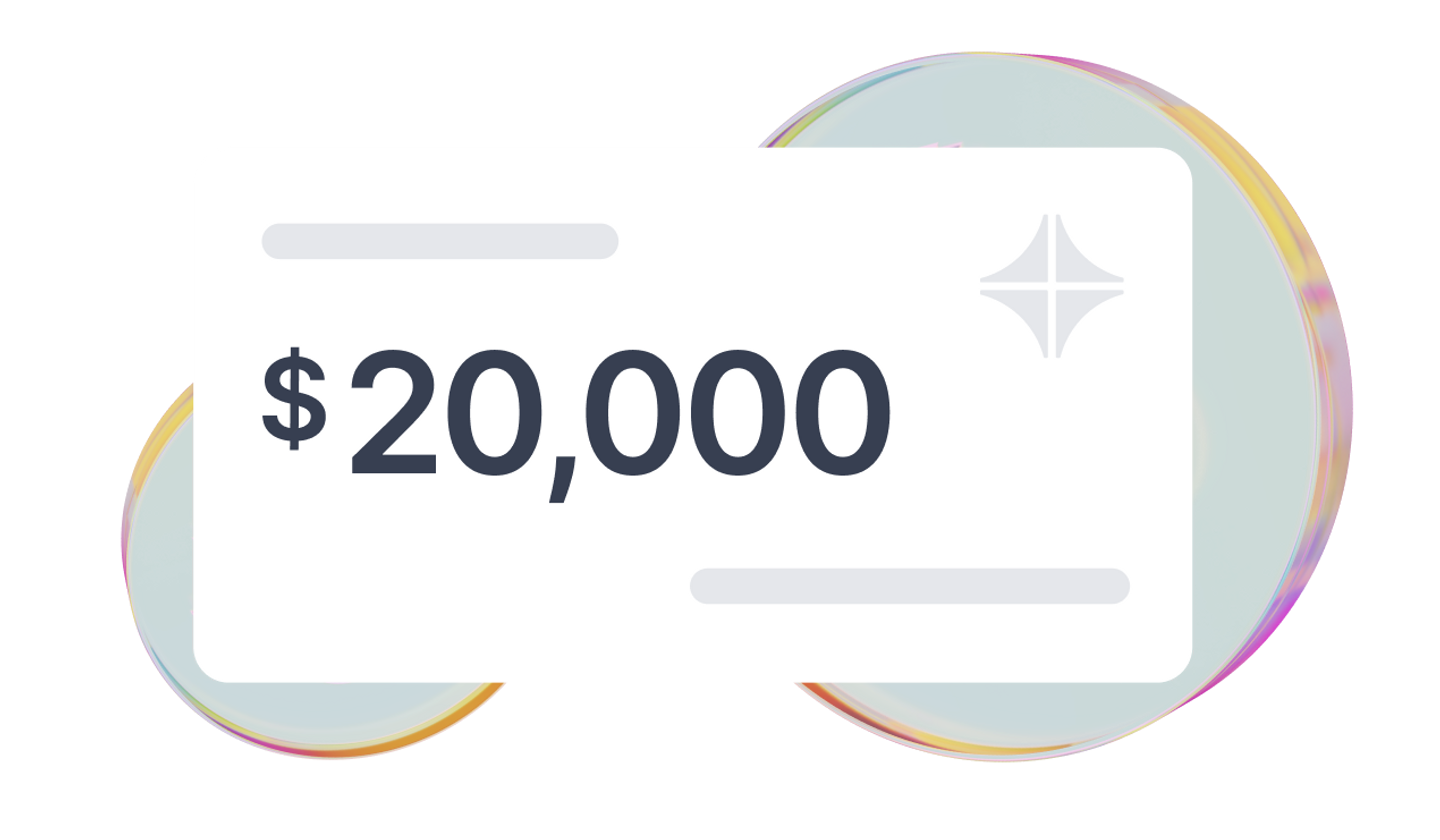
Manage
contracts & payments
Create contracts, pay, and chat with freelancers.
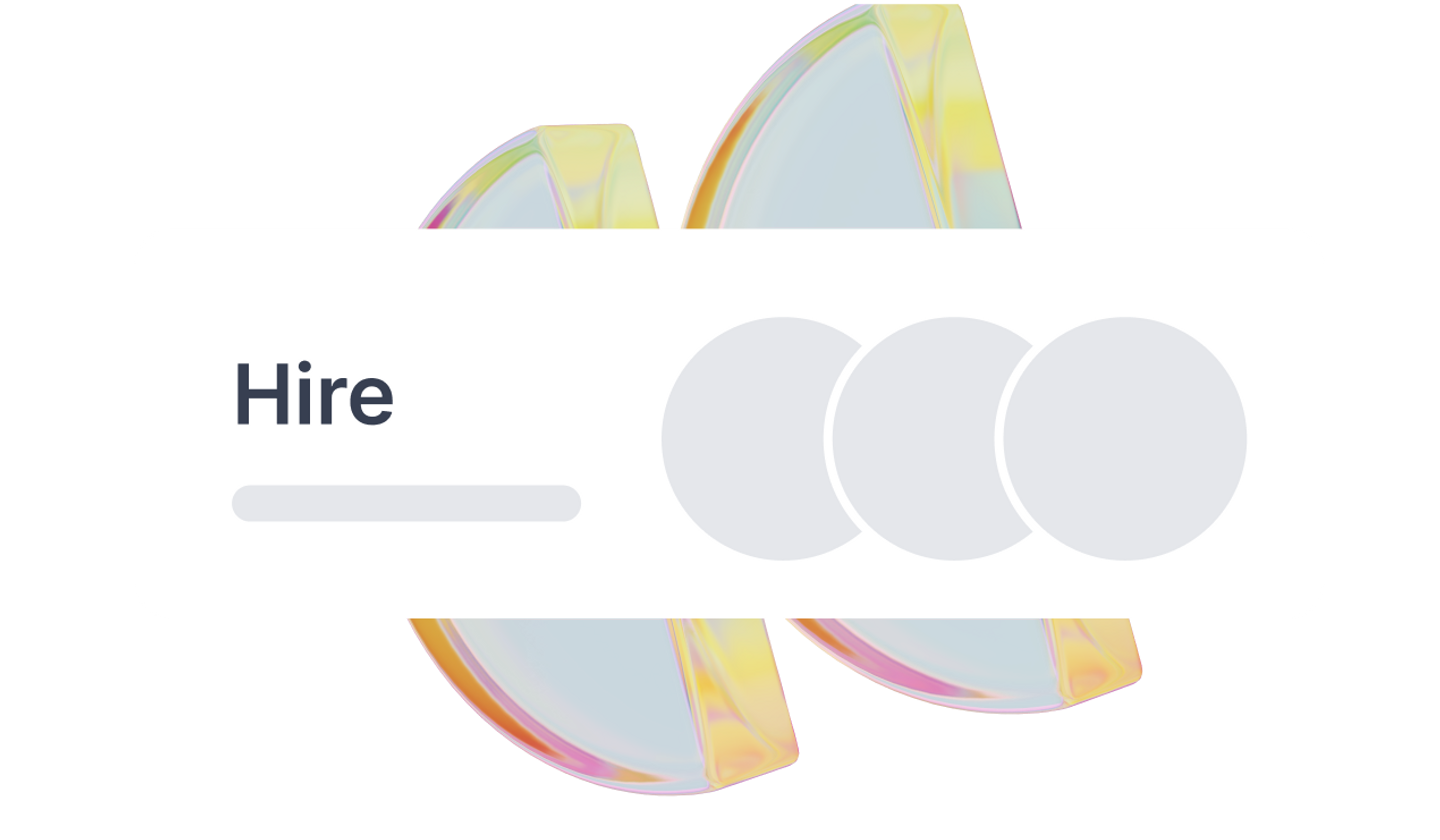
Scale your business
Focus on core business functions by outsourcing.
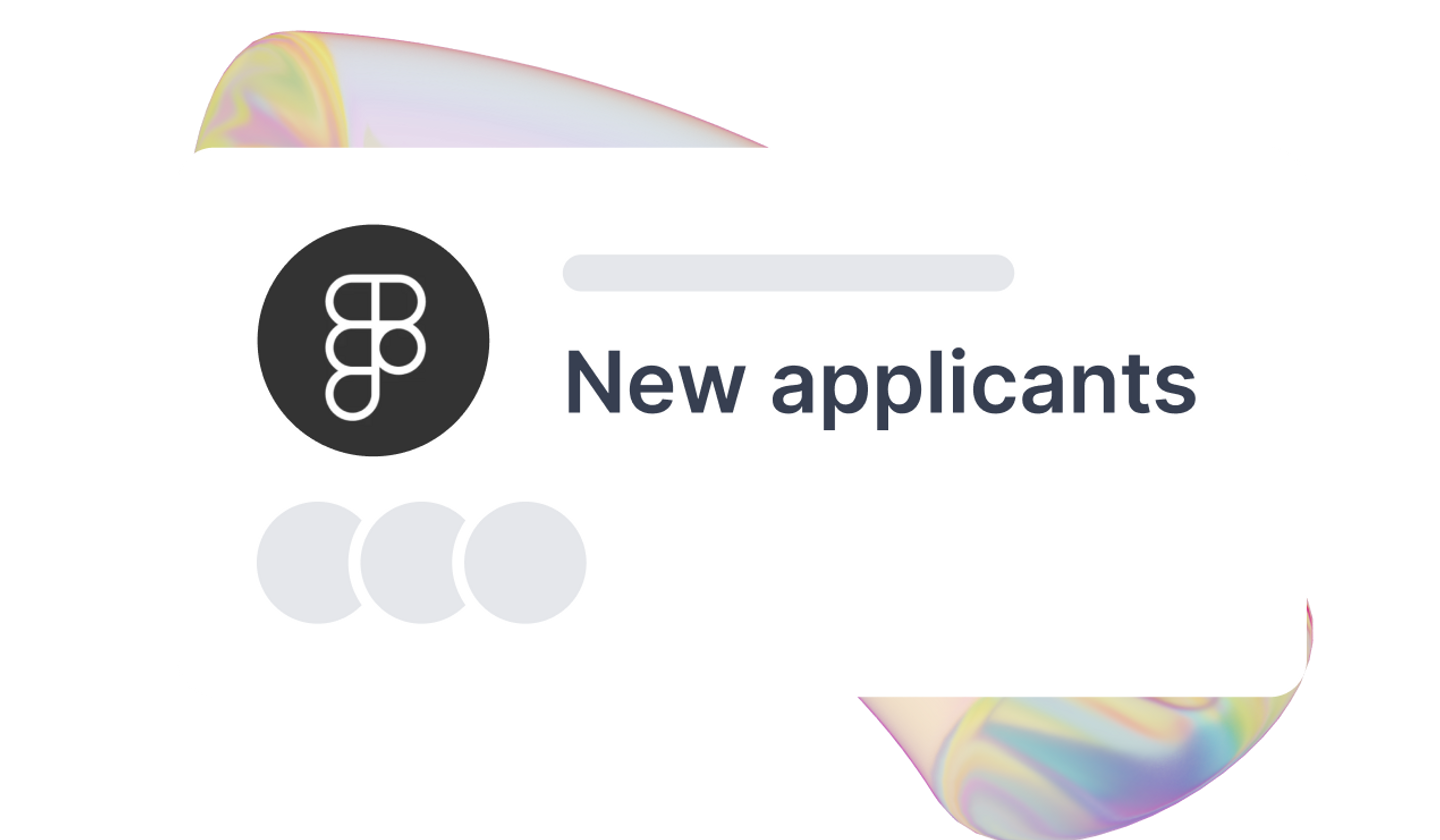
Save time & money
Let our team help you through the process of hiring, all for free.
99% of Clients report having a positive experience on Contra
Why Clients trust Contra
The quality of independents on Contra is top-notch, and the onboarding process is one to model. Being able to speak with a team member to truly understand what we needed as well as offer suggestions of talent in the network was a whole new experience for me.

Love, love, love. Contra is an amazing place to find flexible, creative talent. The process of getting to know independents through their profiles was incredibly easy and fast.

The talent I find on Contra has been excellent. But aside from that, Contraโs community differentiates Contra from other freelancing platforms. I love the responsiveness of the Contra team, and how invested they are in your success. I actually pay freelancers Iโve found outside of Contra through Contra because I love the platform so much, especially that they donโt take a cut of your payment.

I used to waste hours trying to find talent. Switching to Contra was 10x on my time, energy and money as an Entrepeneur and Creator. Lauren was a perfect fit from day one, and I couldnโt have found someone of this calibor without Contra.

I can vouch for Contra that it is a great hiring tool. I shoot my shot and the candidates I keep seeing are incredible.The process took minutes and the support I always get from their team is 10/10.

Freelancers on Contra come across very professionally. They actually seem to read your job posting and have directly relevant skills, which isnโt always the case on most other freelancer sites. On Contra, freelancers who matched with my job seemed to have the relevant skills I was seeking.

Frequently asked
questions
A freelance data engineer is a professional who designs, builds, and manages the data infrastructure of a company. They prepare the 'big data' infrastructure to be analyzed by data scientists. They are responsible for creating and maintaining the algorithms to extract the needed data.
A freelance data engineer is a professional who designs, builds, and manages the data infrastructure of a company. They prepare the 'big data' infrastructure to be analyzed by data scientists. They are responsible for creating and maintaining the algorithms to extract the needed data.
Hiring a data engineer on Contra provides you with top freelance talent without the hassle of middlemen or commissions. You can review their skills, collaborate efficiently, and manage contracts and payments all in one place.
Data engineering is a field of study that focuses on the application and management of methodologies, techniques, and tools for the transformation and integration of data and information in a structured and useful way.
You can post a job detailing your project requirements on Contra. Data engineers who match your requirements can then apply for the job. You can also browse through the profiles of data engineers and invite those you are interested in to apply.
Data engineers often use tools like SQL, Python, Hadoop, and Spark among others. They also use ETL tools and cloud platforms like AWS or Google Cloud.
The cost of hiring a data engineer on Contra averages around $85 to $90 per hour. However, rates can vary based on the complexity of the project, the engineer's experience and skills, and the project duration.
Freelance data engineers are paid commission-free on Contra. They may charge per project, per hour, or per milestone. Payment for each milestone is funded individually at the beginning of the project and held in escrow. The funds for each milestone will only be released after you approve the deliverables for that milestone, or automatically within three days.
How to get started on Contra
Contra for Clients
With Contra, you can discover and hire top-notch talent, manage payments, contracts, and projects, and work the way you want.
Read moreGuide to Discover
This guide will provide the steps to finding your perfect match on Contra using our Discover tool
Read moreHow to Post a Top-Tier Opportunity
If you need an Independent for your next opportunity, you're in the right place. Learn about how you can find your next collaborator.
Read moreHow to hire on Contra
There are three ways to hire on Contra -- weโll go through all three of these in this article and when to use each one!
Read moreBest Practices for Working with Independents
Thinking about hiring an Independent? Here are some tips to maximize your partnership and collaboration
Read moreLearn more on hiring
Explore the Blog
Related articles
Data Engineering vs. Data Science: Key Differences ๐
- Engineering, Dev & IT
QA Engineers: What They Are, and How to Become One โ๏ธ
- Engineering, Dev & IT
How to Become an Audio Engineer in 4 Steps๐
- Design & Creative
How to write a successful job proposal: The freelancerโs guide๐ค
- Remote Working & Freelancing
Guide to Flexible Projects
- Tips for Hiring
Types of Diction to Use in Your Writing: 8 Examples โ๏ธ
- Writing